Anderson Ma 在彭博买方论坛的对话
During the event’s agenda, Anderson engaged in a lively discussion on “How Artificial Intelligence and Quantitative Research Technologies Will Reshape Financial Markets” with four other panelists. The insights and perspectives shared during this discussion received enthusiastic responses from the audience.
Here are the questions he answered in this discussion:
Q: How are you using LLM at your firms at the moment?
A: We currently have multiple LLM (Language Model) assistants, including ChatGPT, New Bing, and Claude, to assist with various tasks such as research, IT coding, and compliance document writing. We will deploy them within web applications, office automation (OA), and instant messaging (IM) software, at the system level to facilitate their use across different languages, system versions, platforms, modes of access, and network environments.
A: Additionally, we will leverage LLMs to assist with fundamental and quantitative research.
A: We primarily use LLM to gather historical data and information in fundamental research. For example, it can retrieve significant financial events from the past, aiding analysts in understanding timelines and event developments quickly. It can also, to some extent, replace search engines by directly fetching relevant information about publicly traded companies, and analyzing news and announcements to understand market sentiment. In quantitative research, we use LLMs to assist with coding to optimize model performance and the accuracy of data predictions. Occasionally, we apply machine learning (ML) and deep learning (DL) during model creation and fitting.
Q: LLM and chatbots are almost always mentioned in the same sentence now. What would you like a chatbot for and what would you prefer to keep human?
A: We primarily use LLM to gather historical data and information in fundamental research. For example, it can retrieve significant financial events from the past, aiding analysts in understanding timelines and event developments quickly. It can also, to some extent, replace search engines by directly fetching relevant information about publicly traded companies, and analyzing news and announcements to understand market sentiment. In quantitative research, we use LLMs to assist with coding to optimize model performance and the accuracy of data predictions. Occasionally, we apply machine learning (ML) and deep learning (DL) during model creation and fitting. In this context, the key issue is the boundary of LLM/Chatbot capabilities. We use chatbots for basic information search, aggregation, and organization, such as arranging the sequence of significant macroeconomic events in history or summarizing the content of company announcements.
A: However, we tend to involve analysts in decision-making and judgment, especially when collected basic information requires inference and analysis to determine which information might ultimately affect stock price trends and lead to trading decisions. Regarding the application boundaries, in the current situation, we find that it is most suitable to assign tasks to these models that are determined by us, are familiar to us, and are repetitive and burdensome. Only in such cases can our human workforce discern whether a task is completed as needed and accurately by the model, rather than being deceived by it. In other words, the model’s capabilities may expand in parallel with the user’s boundaries. We believe LLMs perform exceptionally well in certain areas, especially in text generation and natural language processing, such as searching for materials and collecting information. However, there may be limitations when it comes to tasks that require judgment and analysis. We are uncertain about their accuracy, and, therefore, we are unwilling to rely entirely on LLMs for these tasks.
A: We emphasize human involvement because humans are better equipped to assess the accuracy of information and conduct in-depth analysis. We believe that human judgment and analysis are crucial in decision-making and trading, while LLMs may not be sufficient. This is because, in the realm of investment and finance, accuracy and credibility are paramount, requiring cautious decision-making and judgment.
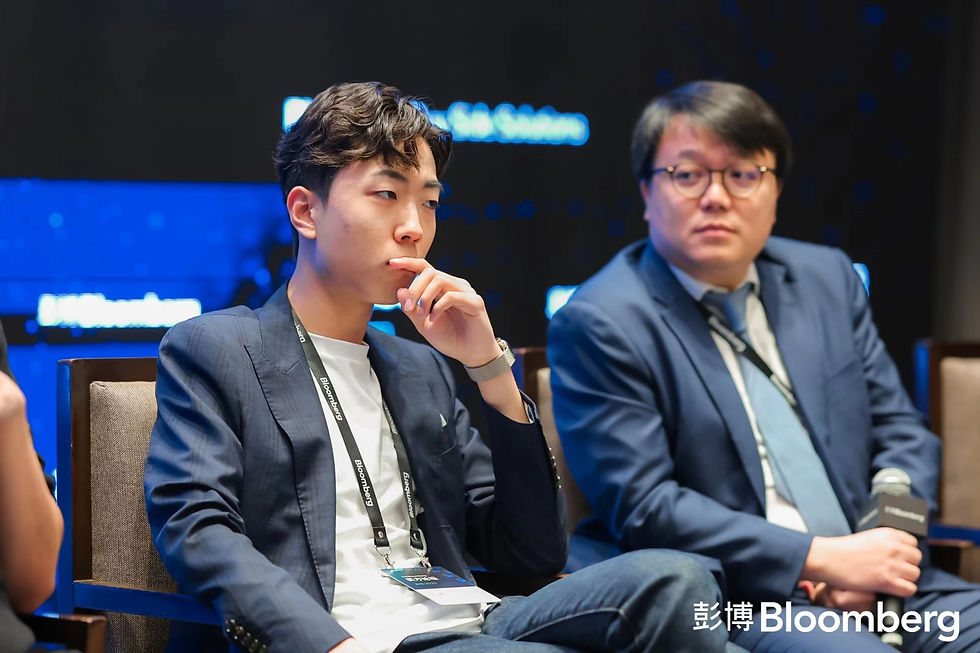
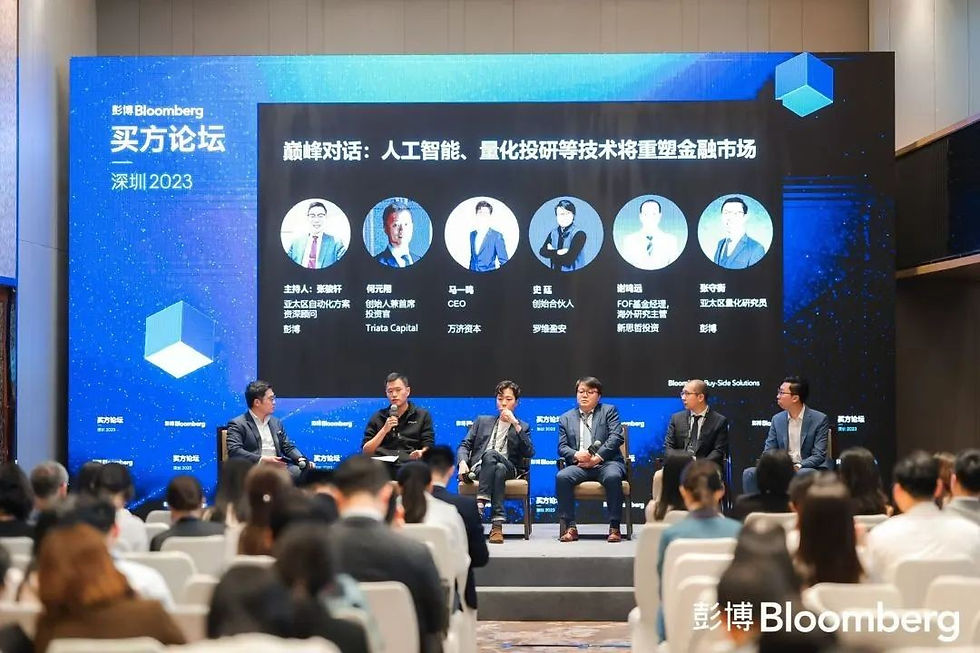
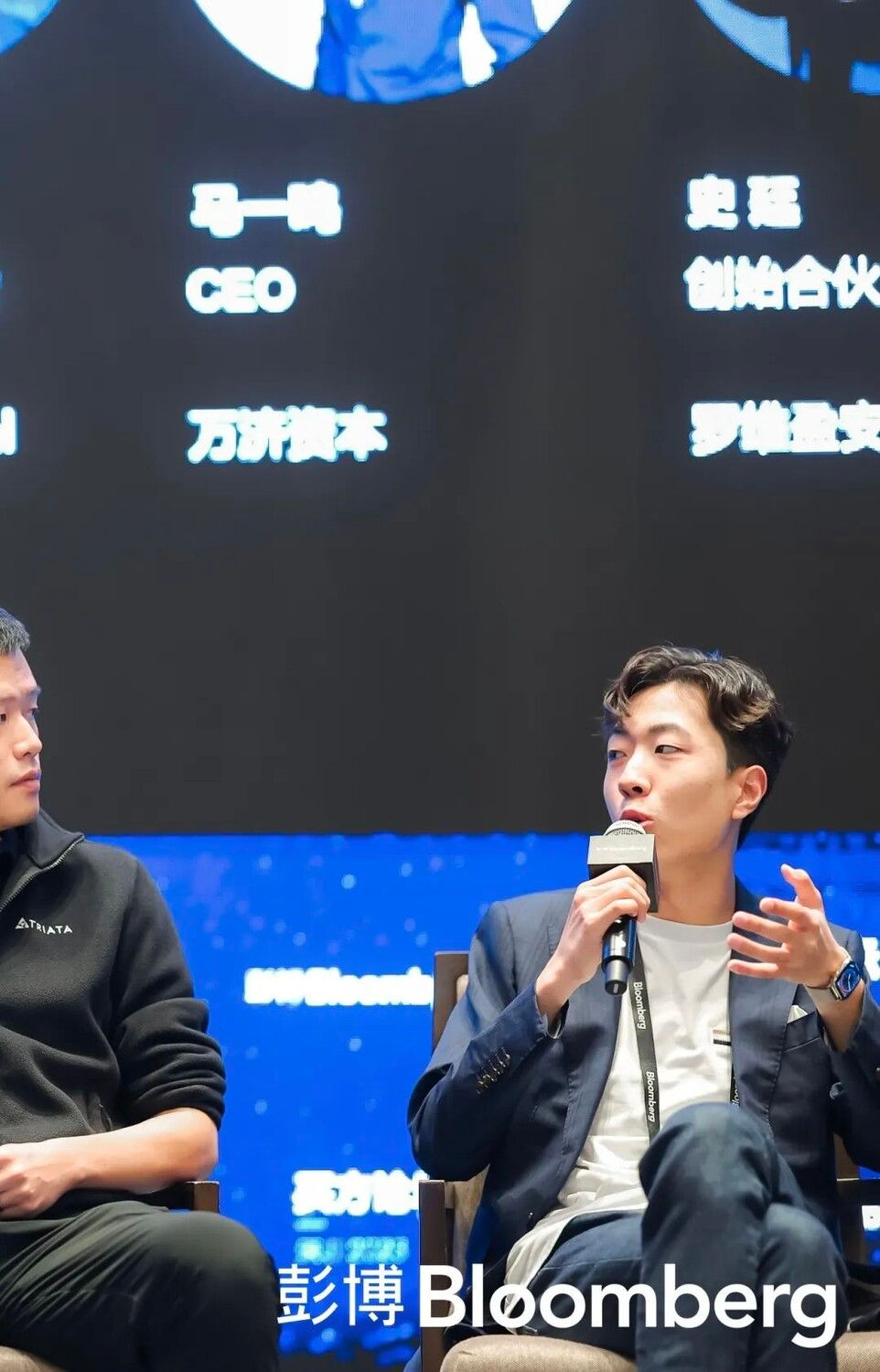